Snowflake runs a sophisticated system of services, and all these systems operate at a large scale, necessitating the execution of software on many dozens of computing resources and millions of processing cores at the same time. Failures on such a grand scale are unavoidably unavoidable. The cloud-based data warehouse is the latest craze. Snowflake has announced the launch of its new project into the financial services industry, while Teradata, a long-time leader in data warehouse management for banks and insurance companies, is attempting to widen its appeal through the use of computer vision implementations.
How Snowflake is important for handling failures in cloud systems?
Whenever customers’ real-time services are hosted on cloud infrastructure, the likelihood that a node may fail is very significant. It should come as no surprise that breakdowns occur more often as computers grow in size and complexity in their architectural design. In this scenario, a long-running program on a cloud server system would suffer numerous pauses and will be pushed back to the place where the calculation was started from the beginning of the previous checkpoints. It is very difficult to deal with these sorts of setbacks.
It is possible to suggest a new method to dealing with failures in a comprehensive manner, rather than waiting for problems to occur and then reacting to these failures. Predictable failure is required for the proactive strategy to be successful. Following the prediction of a failure, the choice is taken to perform a migration from a degrading node to a backup node. Because of this, it is necessary to develop a method for dealing with failures in the public cloud to enhance their dependability, accessibility, and ease of maintenance.
Let’s have a look at what’s underneath:
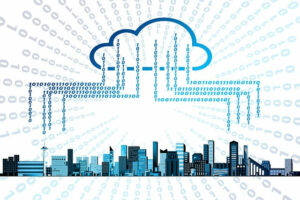
1. Creating analytics solutions are not garbage in or garbage out
The ability to scale Snowflake development is a huge advantage. At the press of a button, you may access almost limitless storage and computing resources. Taking on ever more interesting projects, such as machine learning and deep learning data analytics, is made possible as a result of this. It is much preferable to monitor and verify the data quality before putting it into your processing pipelines. Consequently, the opposite side will provide you with important information that you may put into action right now.
2. Is it possible to do this ETL in stages?
We had to completely rewrite several of our ETLs over the past year to guarantee that all of our data was handled daily. Before the improvements, tasks that were intended to handle full-table refreshes began to spill to the disc because of the high volume of data. Over a short amount of time, some of the datasets increased in size by a factor of five, yet the SLAs stayed the same.
3. Absence of Data Source Information
The contextualize regarding the current data issues, such as missing information, duplication, erroneous data, and misspellings, may substantially impair data integrity. When migrating the data from one cloud to another, companies must be aware of the data that is being transferred. This, however, is a time-consuming and intimidating procedure to do. It has the potential to entangle a variety of resources that might have been put to better use in other company activities.
4. Data Analysis Done Incorrectly
Certain information in the data may be concealed as a consequence of the computer specification since there are no particular fields in the system to store this information. Because of the absence of certain variables, the data will not flow into the new system correctly. As a result, before migrating data to a new infrastructure, companies must do a thorough data analysis of their existing data. As a result, these are the difficulties that may arise during the data transfer.
5. Identifying and troubleshooting unsuccessful queries
Incorporating sufficient debug information into the design of an excellent production network is one of the basic principles of creating a system that can be used to examine production problems quickly and easily. It may be argued that the auto-retying of requests adds a layer of complication to the process of troubleshooting errors.
Through the use of numerous compute instances, the architecture is designed to make it easier to triage errors by chaining query execution attempts together. Designers are capable of creating a comprehensive query-execution lifetime chronology that includes all of the occurrences that are relevant to them.
Differences between Traditional Data Warehouses and Cloud Data Warehouses
In its most basic form, a conventional data warehouse is an infrastructure for organizing, storing, and retrieving ordered data that is housed on-premises in a data center controlled by the company whose data is included inside it. It has a limited size and strength, and it is controlled by the organization that created it.
A cloud data warehouse is a scalable amount of storage and computing capacity that is housed inside a much larger public cloud data center and that can be accessed and controlled entirely via the internet. Storage space and computing power are simply leased resources. The geographical space of the data is generally immaterial, except for nations and/or businesses whose laws require that their data be kept in the very same country as the data source.